10 things about Digital Twins in agriculture
“Richard Tiffin, our Chief Scientific Officer, and I were recently recounting the number of conversations we’re having about Digital Twins. As a result, we wrote this article to answer 10 common questions people have about Digital Twins in agriculture. Enjoy!”

Dr Matthew Smith
CPO, Agrimetrics
Digital Twins are becoming more popular. Gartner estimates that by 2021, half of large industrial companies will use Digital Twins, resulting in a 10% improvement in operational effectiveness.
Agriculture is no exception. Dutch firm Connecterra have created Digital Twins of dairy cows. Meanwhile, John Deere is analysing real-time data from their machinery in the cloud; predictive maintenance being one of their objectives. Digital Twins have a natural synergy with Internet of Things (IOT) technologies, which over time will accelerate their rise. As IOT proliferates within agriculture, Digital Twins will follow.
This article will answer some basic questions about Digital Twins in agriculture.
What are Digital Twins?
A Digital Twin is a sufficiently realistic digital representation of a real-world thing. They can be used to monitor the ‘thing’ remotely. For example, a Digital Twin of a cow could alert the farmer to poor health, without that farmer having to examine the animal.
To be more specific, a Digital Twin needs to be:
- Individual: It must represent a specific thing, e.g. “Daisy the cow” rather than a generic cow.
- Near real-time: This also means that the Digital Twin should be “always on”; available for as long as its real-world counterpart exists.
- Data-informed: It must be updated via a digital measurement of the real-world thing, e.g. a soil moisture meter or a regular satellite observation.
- Realistic: The Twin must be a sufficiently realistic surrogate for the real-world thing.
- Actionable: Information from the real-world twin must have the potential to lead to an action.
How are they possible?
A confluence of technological advances have made Digital Twins possible: improved monitoring via sensors and devices, ubiquitous connectivity and computation, and improved systems understanding and modelling via machine learning.
Consider a Digital Twin of a field. We can acquire field-status updates from sensing systems: satellites, drones, weather stations, tractor mounted sensors, soil probes. We can also collect data on historical soil information, slope and aspect. Management data is another key information source, such as what crops were planted when and fertilisation dates; these are often recorded digitally on systems such as Gatekeeper and Muddy Boots.
Modelling Uncertainty
All these data sources provide a wide range of signals, but it will not be comprehensive. Gaps will exist due to a lack of available data or because that parameter cannot be measured directly. For example, it would be difficult to directly measure the total digestible dry matter available in a field of grass.
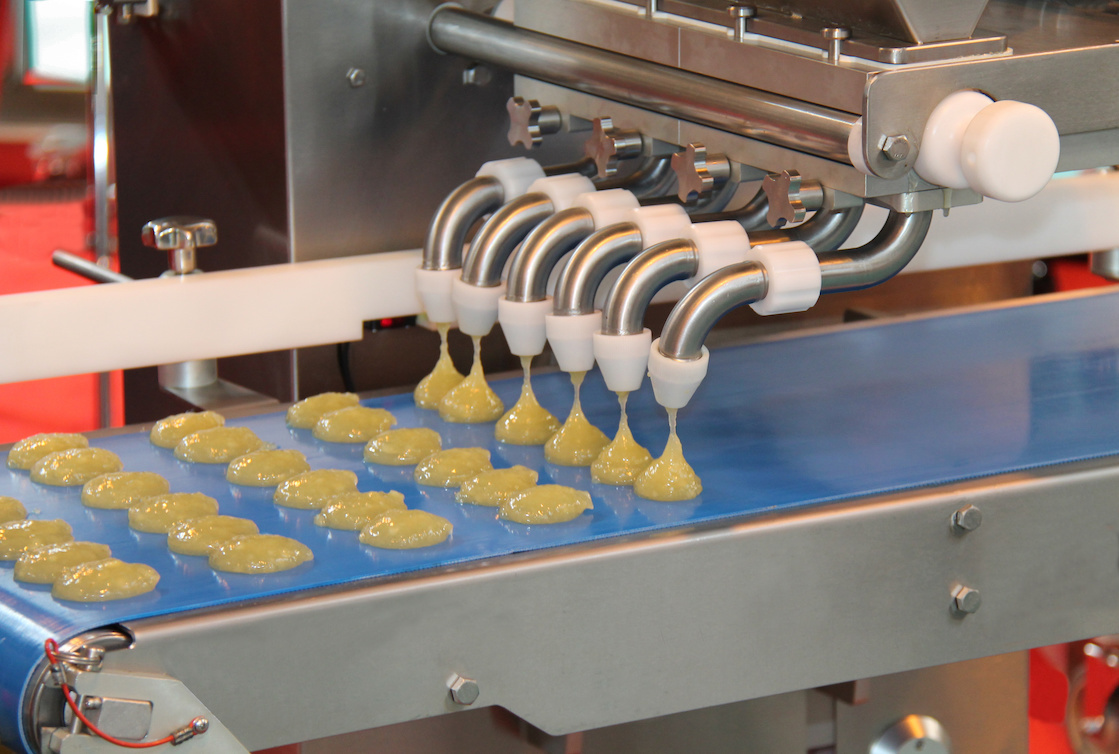
Smart Factories in other industries are starting to deploy Digital Twins. Amongst other things, this has enabled the rise of predictive maintenance
However, it’s often possible to infer these measurements using other information. Thus, Digital Twins generally include a computational modelling system that fills these gaps. These systems must go beyond generic models, e.g. grass growth, and accurately represent all of the relevant processes taking place in the field: chemical, physical and biological. The model must reflect the true mix of grass species in the field. And it must be able to learn. They must also simplify access to incredibly complex information for the benefit of the decision maker.
A Digital Twin doesn’t have to be sophisticated. They could represent a very narrow aspect of the real-world, e.g. the fluid quantity in a milk tank. The key is that they are sufficiently realistic.
How are they different from models?
Models use algorithms to simulate and sometimes predict systems. This is not dissimilar to processes Digital Twins use to fill informational gaps. However, though Digital Twins do incorporate such models, they do much more.
Individual models typically improve our generic understanding of a system or make generalised predictions. They are rarely used to accurately represent the status of a specific thing. Often, they are wrong.
“
Because they are generic, there is a recognition that models are always wrong.
— Dr Matthew Smith, Chief Product Officer, Agrimetrics
Multiple models are sometimes combined to give an indication of how wrong they are. For example, this can be done by examining how the different model predictions vary in order to understand how predictions are influenced by their formulation.
In a Digital Twin, models are meant to reflect real-world uncertainty. They are used for highly specific purposes; their output is seen within the context of dozens – or perhaps millions – of other data points and outputs. This gives rise to the possibility that the model is not wrong.
Additionally, Digital Twins are designed to shield the user from unnecessary technical complexities. They are intended to expose information about the real-world in a way that enables actions to be easily taken. They are also intended to be “always on” and “real time”: the status of the Twin should always accurately reflect the current status of the real thing, regardless of when you consult it. Neither of these attributes are expectations of models more generally.
How do you interact with a Digital Twin?
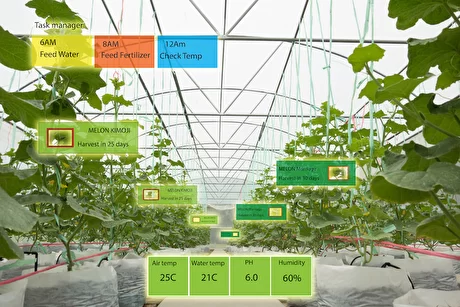
Digital Twins are typically accessed through a virtual interface, which displays information about the status on the ‘thing’
Interaction with a Digital Twin would usually be through a visual interface on a phone, tablet or computer. This would let you see information about the status of the thing, its history (e.g. an ewe’s health history) or its predicted future (e.g. a crop growth forecast). You are also likely to be able to interact with the real-world system through the Digital Twin, such as switching on an irrigation system.
In the future you might also be able to run what-if scenario experiments, e.g. If I added plant growth regulator now what would happen?
A key property is that a user is hidden from almost all of the complexity. The inner workings of the Digital Twin are invisible. The user is exposed only to those properties they would normally be exposed to when dealing with the real-world counterpart. However, there is the added bonus that they can do things with the Digital Twin that they couldn’t do in the real-world.
Where am I likely to see Digital Twins in Agriculture?
The beginnings of Digital Twins are already appearing. Livestock monitors are enabling remote monitoring and modelling of aspects of animal health. Field information systems are reporting on the status of fields and crops to inform management decisions. Remote monitoring of farming machinery such as tractors enables detection or even pre-emption of problems.
For these, a subset of the real-world thing is monitored and modelled sufficiently realistically that the live update has real meaning about the status of the system. However, these Digital Twins have a restricted scope: you can only consult the Digital Twin for a cow about specific aspects of its health and not, for example, about its antibody levels.
In other industries, such as the Digital Twin of a building in engineering, Digital Twins can cover a broader set of properties because both the monitoring is more complete (more sensors) and the functioning of the system is better understood (physics-based models). In agriculture we are still waiting for understanding and monitoring ability to improve sufficiently to enable comprehensive digital surrogates.
“
Digital Twins of fields, livestock and machinery are set to improve as IOT tech proliferates, and centralising platforms – such as The Data Marketplace – gain more users.”
— Dr Matthew Smith, Chief Product Officer, Agrimetrics
You are most likely to see Digital Twins at the convergence of need and practicality (some things are easier to digitise than others). Twins of supply chains are being developed in other industries and agri-food is sure to follow. The disruption caused by Covid-19 is a significant motivator, yet even before this pandemic there were loud demands for greater traceability and reduced waste.
Digital Twins of farms and agricultural landscapes could also be on the horizon.
What are the use cases?
Livestock Management
Herd management is a classic use case for Digital Twins. Sensors on or in the cow (e.g. accelerometers and boluses), at feeding and milking stations and elsewhere (e.g. weighbridge) collect a continuous stream of data. This information is processed through to provide an indication of the milk or meat production efficiency and animal health. The farmer can then act accordingly. While the action taken might not be novel, the information derived from the Digital Twin will enable earlier – and possibly more effective – intervention, offering the potential to improve animal outcomes.
Arable Farming
In an arable context, farm managers would routinely consult Digital Twins of their fields. This would report on the current soil, water, crop and other properties. They would also support future projections of those properties. This information would be used to inform management actions, such as addressing shortfalls in production, the risk of lodging, anticipated over or under production and crop rotation plans.
Indoor Farming
One of the most promising landscapes for Digital Twins are controlled growing environments, i.e. indoor farming, aquaculture and under glass. In these cases, capturing sufficient data and working out what is happening is easier analytically. A producer would regularly consult the Digital Twin to keep track of production, issue interventions (probably remotely) and create a future management schedule.
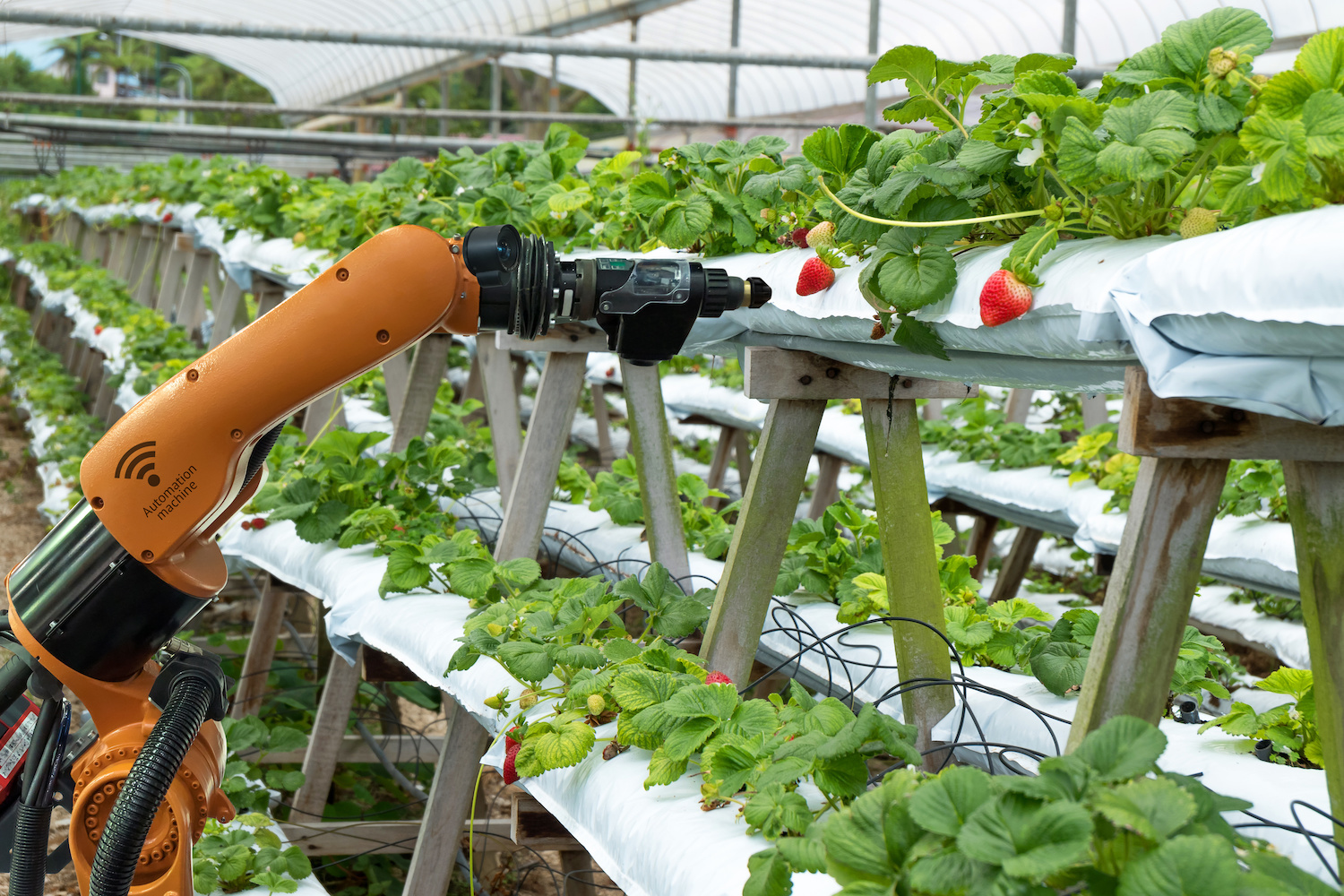
Indoor farming is a promising prospect for early Digital Twins, due to the controlled environment and the relative high-value of their produce
Improving Sustainability
Digital Twins could be used to provide assurances about the Natural Capital of an agricultural landscape. Sensors providing evidence would need to be widely distributed, which is why satellite data is highly promising. From an agricultural perspective, Digital Twins would enable the tracking of carbon, biodiversity, pollinator and water catchment services. It would tell us whether they are changing and, importantly, whether those changes are caused by us.
At some level, each of these use cases can be met now, given the right investment in sensors, models and interfaces. However, these would not necessarily meet our definition of a Digital Twin because they would be reliant on generic crop, livestock and other models. To be a full Digital Twin, these models must learn from observation and adapt to become a bespoke model for the twinned entity.
Why does this matter?
Digital Twins matter because they enable systems to be managed more effectively: saving time, costs, improving sustainability and attracting higher premiums for produce.
They enable users to make management decisions about things without them having to be physically present: this is both for short term responsive actions and longer-term planning. Real time information about animal health could be consulted and responded to by vets, the weekly field management plan could be more easily constructed by having the digital representation of all the farms fields. The Digital Twin of a farm could be used to provide transparency to an end consumer about the conditions of that farm to justify a higher premium.
What are the risks?
Effective Digital Twins are likely to be expensive in many cases; they will require sufficient financial justification. Mapping of the systems that influence the ‘thing’, deploying and connecting sensors, transforming the data, and running the digital system will add up.
“
A key risk of developing Digital Twins in agriculture is that the benefits they confer are less than the cost of developing and deploying them in the first place.
— Dr Matthew Smith, Chief Product Officer, Agrimetrics
A perceived risk is that decision makers, i.e. farmers, become separated emotionally or intellectually from the real-world system. It’s feared that this could lead to a failure to detect and respond to details that would have been seen in reality. Particularly relevant for livestock farmers is the worry that over-focus on digital performance metrics could reduce empathy.
Fortunately, these issues are being studied. Results have shown that Digital Twins can successfully augment decision making, with few negatives. By enabling users to make decisions faster, Digital Twins may actually free up time to focus on facets not represented by the Twin.
Who can deliver them?
Digital Twins require an expensive combination of experts to develop them: domain experts, sensor and IoT experts, machine learning and AI experts and several more. As a result, the creation of Digital Twins cannot be undertaken lightly.
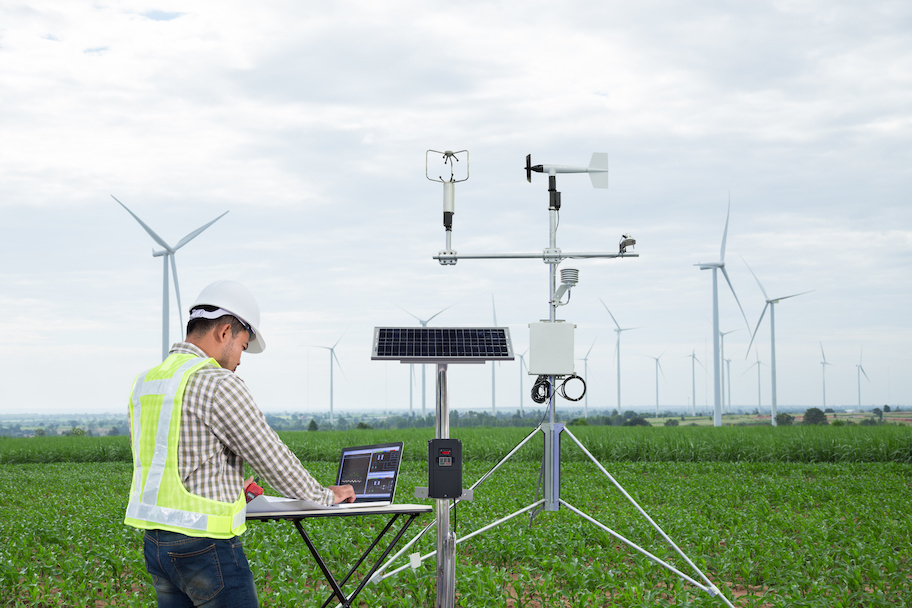
Due to their cost and complexity, he first Digital Twins are likely to appear in organisations with large R&D budgets and significant ROI potential
On top of that, it requires the identification of a valuable enough set of use cases: Digital Twins must demonstrate return on investment (ROI). Not least because long term running and maintenance costs, though substantially less, are still not insignificant.
Digital Twins are likely to be developed by high-tech start-ups or advanced agribusinesses with significant R&D budgets.
As a result, Digital Twins are likely to be delivered by start-ups, such as Connectra, with the correct combination of expertise, technology and funding. Public Sector bodies and large agribusinesses with innovation budgets are another likely originator; John Deere is actively innovating in this space, as is BASF through its Xarvio platform. However, these organisations are likely to require support – this will provide opportunities to well-placed start-ups.
The more rapid development of Digital Twins in other industries, such as Smart Cities and Smart Factories, raise the possibility that a delivery capability could come into agriculture from elsewhere, provided they can acquire the domain understanding.
What’s the future of Digital Twins in Agriculture?
Digital twins are inevitable in high value production systems in agriculture: indoor production, fresh produce, and high value livestock. The beginnings of these systems are already starting to emerge, and a true digital twin is likely just a couple of years away.
They also seem likely to emerge for the continuous monitoring and reporting system for the wider agri-landscape: ELMs and the growing emphasis on carbon and natural capital accounting will be key drivers here. However, this will take significant time due the technical challenges.
A key future R&D direction is to identify ways to reduce the overall cost of developing and deploying Digital Twins. If successful, such research could bring this capability to many more applications.
About Agrimetrics
Agrimetrics is the food and farming sector’s Data Marketplace. We enable organisations to safely share and monetise their data, whilst making it easier for data-consumers to access the information they need. Our goal is to help create a more productive and sustainable food system by enabling next-generation solutions as quickly and affordably as possible.
We are one of four centres for agricultural innovation founded with an initial investment from Innovate UK. Our founding partners are NIAB, SRUC, Rothamsted Research and The University of Reading. We have strategic partnerships with Airbus and Microsoft and are a participant in Microsoft’s prestigious AI for Earth programme.
The four centres
The Agri-Tech Centres are a unique collaboration between Government, academia and industry created to drive greater efficiency, resilience and wealth across the agrifood sector. A £90m investment from the UK’s strategic innovation agency (Innovate UK) is enabling the Centres to harness leading UK research and expertise as well as build new infrastructures and innovation. They include CHAP (Crop Health and Protection), CIEL (Centre for Innovation Excellence in Livestock), Agri-EPI (Engineering and Precision Technologies), and Agrimetrics.
Other articles that might interest you
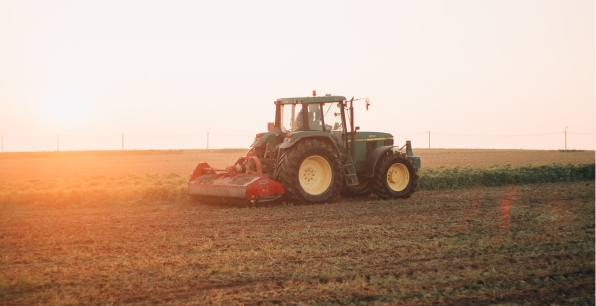
AI identifies crops from space with 90% accuracy
Agrimetrics launches CropLens AI, a proprietary algorithm that identifies crop types from space.
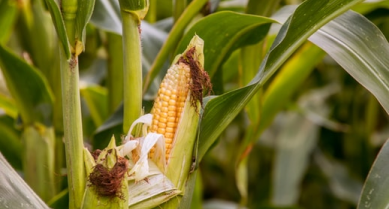
ClearSky: Cloud free satellite data for agriculture
ClearSky's artificial intelligence removes a major barrier to the efficacy of satellite
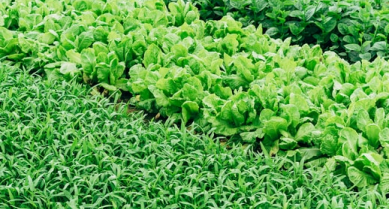
ClearSky: Cloud free satellite data for agriculture
ClearSky's artificial intelligence removes a major barrier to the efficacy of satellite
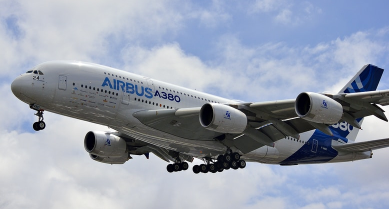
Agrimetrics partners with Airbus to reduce cost
Crop Analytics, a crop and field analytics package from Airbus, is now available at a new, lower price.