Measuring Nature: Why it is important and what it can do to help your business – Part 7
What is Machine Learning?
How does it work?
How can it help us understand at scale?
We have previously looked at positioning as a complimentary technology to earth observation and there are others that improve and enhance the images created from space as well. A rapidly developing area that could see a step-change in the way earth observation data is created and used in agricultural applications is Machine Learning.
The Imitation Game, later renamed to the Turing Test after its inventor Alan Turing, is a measure of how closely a machine can exhibit the behaviour of a human being. When Turing first introduced this idea in his 1950 lecture with the words; “Can machines think?”, it was considered closer to science fiction than fact. Today, many people carry mobile phones in their pockets that will only work once they have recognised their owners’ faces.
Whilst ‘Artificial Intelligence’ is a term that covers the whole gamut of using computers to mimic human behaviour, ‘Machine Learning’ focuses on imitating and ultimately improving the way data is implemented through the use of self-learning algorithms.

The term ‘self-learning’ means that the feature-recognition algorithms, which is to say, the processes that can automatically recognise barns, field boundaries or the types of crops that are contained within the image, will get better the more images they are able to ‘look at’.
In Part 6 of Measuring Nature, we looked at where earth observation data can be purchased and discovered that there is an ever-expanding number of sources, producing ever more images from space. The Copernicus satellite data repositories alone hold well over 30 petabytes of data, and each day that total increases by more than 25 terabytes (visit the OpenAccess Hub to explore this amazing library).
These are almost unimaginably large numbers (one petabyte equals one million gigabytes) and it is simply impossible to find and employ enough remote sensing analysts to do the work of turning these images into useful and usable information.

So, machine learning needs as much data as possible if it is to do a good job and there is a vast amount of earth observation data being created everyday that the needs to be processed. It isn’t hard to understand why the use of machine learning is increasing so rapidly within the remote sensing industry.
At Agrimetrics, we have used Machine Learning ourselves to develop our UK Field Boundaries which provides an outline of UK fields. By utilising these techniques and capabilities we can develop solutions at scale and with greater accuracy and efficiency.
Other articles that might interest you

AI identifies crops from space with 90% accuracy
Agrimetrics launches CropLens AI, a proprietary algorithm that identifies crop types from space.

ClearSky: Cloud free satellite data for agriculture
ClearSky's artificial intelligence removes a major barrier to the efficacy of satellite
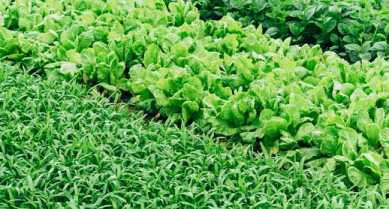
ClearSky: Cloud free satellite data for agriculture
ClearSky's artificial intelligence removes a major barrier to the efficacy of satellite
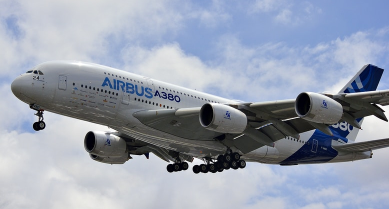
Agrimetrics partners with Airbus to reduce cost
Crop Analytics, a crop and field analytics package from Airbus, is now available at a new, lower price.